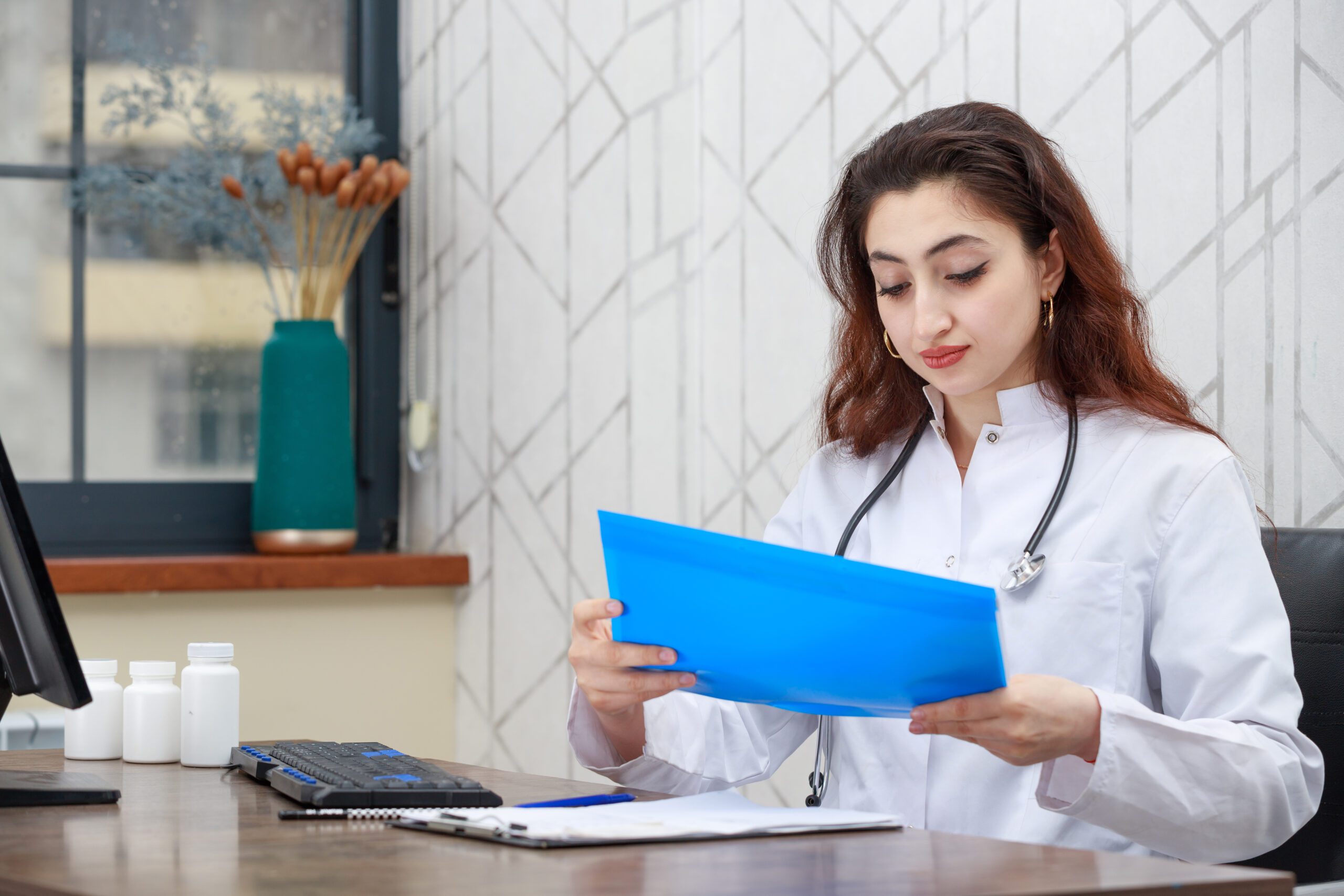
Predictive Analytics in Revenue Cycle Management Explained
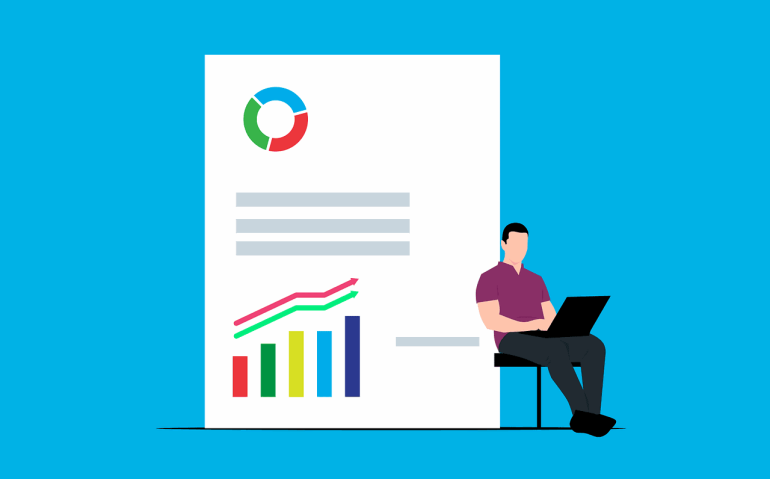
Revenue cycle analytics stands as a transformative tool, employing predictive data analytics to forecast trends, identify potential bottlenecks in the revenue cycle, and suggest actionable strategies for improvement. By harnessing the power of predictive analytics, healthcare providers are now better equipped to navigate the complexities of claims processing, denial management, and overall financial health, making it a cornerstone in the pursuit of operational excellence and financial stability.
This article delves into how predictive data analytics is revolutionizing revenue cycle management by offering insights into understanding predictive data analytics in healthcare, enhancing revenue cycle performance, steps for successful implementation, and overcoming common challenges. It will also highlight case studies and success stories, underscoring the tangible benefits that facilities have realized by integrating revenue cycle analytics software and adopting a data-driven approach to RCM reporting. Through this exploration, readers will gain a comprehensive understanding of the ins and outs of predictive revenue strategies and how they contribute to a more efficient, profitable healthcare system.
Understanding Predictive Data Analytics in Healthcare
Predictive analytics in healthcare harnesses current and historical data to empower healthcare professionals in making more efficient operational and clinical decisions. This analysis aids in predicting trends and managing disease spread, enhancing the overall quality of patient care. The scope of healthcare data is vast, encompassing administrative records, health surveys, disease registries, and electronic health records (EHRs), making it a pivotal tool across the healthcare industry.
The transformation of raw healthcare data into actionable insights significantly impacts various areas such as clinical research, disease prediction and prevention, and the development of new treatments. Moreover, predictive analytics facilitates more accurate diagnoses and the automation of hospital administrative processes, which in turn leads to more successful medical outcomes and a more efficient calculation of health insurance rates.
One of the paramount benefits of predictive analytics is its ability to provide comprehensive access to diverse data types—medical histories, demographics, economic data, and comorbidities. This data richness enables healthcare providers to make informed, data-driven decisions that significantly enhance patient care. For instance, by analyzing past patient data, machine learning algorithms can tailor treatment plans that are most likely to succeed for individual patients, moving away from the traditional one-size-fits-all approach.
Furthermore, predictive analytics is instrumental in population health management and chronic disease management. It allows healthcare organizations to identify similar patient profiles within a population, aiding in the management of disease outbreaks and the enhancement of survival rates through timely interventions. It also plays a crucial role in predicting patient risks, thereby enabling early interventions to prevent more severe health issues, particularly in patients with chronic diseases.
In addition to healthcare, predictive analytics has proven its utility in industries like manufacturing and telecommunications for preemptive maintenance. Similarly, in healthcare, it can predict equipment failures, allowing for scheduled maintenance that minimizes disruption and maintains continuous patient care.
The integration of predictive analytics into healthcare not only optimizes patient care but also reduces costs by minimizing unnecessary treatments and hospitalizations. It also aids in predicting staffing needs, which further controls operational costs. By leveraging predictive analytics, healthcare providers like Wenour can enhance operational efficiency and patient outcomes, ensuring high-quality care at reduced costs.
How Predictive Data Analytics Enhances Revenue Cycle Management
Predictive Analytics for Claim Submission
Predictive analytics significantly enhances the accuracy and efficiency of claim submissions in revenue cycle management. By employing advanced algorithms and machine learning, healthcare providers can predict the likelihood of claim approval and preemptively address potential issues. This proactive approach reduces the incidence of claim denials and accelerates the reimbursement process, thereby optimizing cash flow for healthcare organizations like Wenour.
Proactive Denial Management
Proactive denial management is a critical aspect of revenue cycle management where predictive analytics plays a transformative role. By analyzing historical data and payer guidelines, predictive models can identify patterns that lead to denials. This insight allows healthcare providers to implement targeted interventions such as improved documentation and staff training, significantly reducing the occurrence of denials and enhancing financial outcomes.
Precise Patient Billing
Predictive analytics also contributes to more accurate and personalized patient billing. By analyzing past billing data and patient payment behaviors, healthcare providers can tailor billing practices to meet the specific needs and financial capabilities of each patient. This not only improves patient satisfaction but also reduces billing errors and enhances the overall efficiency of the billing process, leading to better management of the revenue cycle.
Steps to Successfully Implement Predictive Data Analytics
Understanding Your Data
The initial step in successfully implementing predictive data analytics involves a comprehensive understanding of the available data. Healthcare organizations need to assess the quality, volume, and variety of data they possess. This includes reviewing historical patient records, treatment outcomes, and financial transactions to ensure the data is accurate and complete. By establishing a robust data foundation, organizations like Wenour can effectively utilize predictive analytics to anticipate patient needs and improve service delivery.
Building a Skilled Team
Implementing predictive data analytics requires a team with specialized skills in data science, healthcare analytics, and machine learning. Recruiting individuals who are proficient in these areas is crucial for interpreting complex data sets and developing accurate predictive models. Training existing staff and investing in continuous learning are also vital steps to keep pace with the evolving nature of healthcare analytics. This skilled team will be responsible for translating raw data into actionable insights that can drive decision-making and strategic planning.
Leveraging Advanced Tools
To harness the full potential of predictive data analytics, healthcare providers must invest in advanced analytical tools and technologies. These tools should offer capabilities such as real-time data processing, advanced machine learning algorithms, and intuitive reporting interfaces. For instance, revenue cycle analytics software can help organizations like Wenour streamline claims processing and enhance financial performance by providing detailed insights into every stage of the revenue cycle. Utilizing these tools effectively allows healthcare providers to improve operational efficiencies and patient outcomes by making data-driven decisions swiftly.
Key Challenges and Solutions in Predictive Data Analytics Implementation
Overcoming Data Silos
Data silos pose a significant challenge in predictive analytics, as they prevent a unified view of data across an organization. Breaking down these silos is crucial for enhancing collaboration and decision-making. Integration solutions enable seamless data flow across various healthcare systems, improving data accessibility and care coordination. By centralizing data and adopting technologies like cloud-based platforms, healthcare organizations like Wenour can ensure real-time access and better data security, fostering a more collaborative and efficient environment.
Ensuring Data Quality and Integrity
The success of predictive analytics heavily depends on the quality and integrity of the data used. Ensuring data completeness, accuracy, and consistency is vital for reliable predictive models. Healthcare providers must implement robust data quality management practices, including automatic data quality checks using tools like Informatica Data Quality or IBM InfoSphere Information Analyzer. These practices help in maintaining high data integrity, crucial for healthcare providers like Wenour to make informed decisions and enhance patient care.
Addressing Regulatory Compliance
Regulatory compliance is a critical aspect of predictive analytics in healthcare. Organizations must adhere to standards such as HIPAA in the US, which mandates strict data privacy and security measures. Compliance ensures the protection of patient data and builds trust in the healthcare system. Healthcare providers must implement comprehensive security measures like encryption and access controls, and stay updated with regulatory changes to avoid legal liabilities and ensure patient safety.
Case Studies and Success Stories
Geisinger Health System
Geisinger Health System has leveraged predictive analytics to significantly advance its capabilities in managing sepsis, a critical area that affects numerous patients annually. By collaborating with the IBM Data Science Elite team, Geisinger developed a sophisticated predictive model that utilizes extensive data from the health system’s electronic health records. This model is designed to identify at-risk patients early and facilitate the creation of personalized care plans, potentially increasing patient recovery rates.
The predictive model integrates clinical data from a decade’s worth of de-identified patient records to forecast patient mortality during hospital stays or within 90 days post-discharge. This approach has enabled the identification of key clinical biomarkers that predict higher mortality rates in sepsis patients, assisting in more targeted and effective treatments.
Moreover, Geisinger’s commitment to using data-driven strategies extends to its revenue cycle management. The health system partnered with VisiQuate to enhance the efficiency of its revenue cycle operations. This collaboration aims to improve yields, reduce time to revenue, and lower the cost of collections by employing predictive analytics to segment receivables and optimize claim submissions.
Intermountain Healthcare
Intermountain Healthcare has embraced data analytics to improve patient care and reduce operational costs across its extensive network of hospitals and clinics. A study highlighted by MIT Sloan Management Review showcases how systematic data review has informed better treatment protocols and led to cost reductions by identifying the most effective care pathways.
The integration of an advanced electronic health records system has been a cornerstone of Intermountain’s strategy. This system supports a data-rich environment that enhances patient care by providing comprehensive data access to healthcare providers. This integration has facilitated more informed decision-making and enabled a more personalized approach to patient care.
Intermountain’s commitment to continuous improvement and innovation is evident in its efforts to reduce waste and enhance efficiency. The organization’s initiatives in process improvement have significantly reduced the utilization of clinical resources, such as ventilators, resulting in substantial cost savings and improved patient outcomes.
Furthermore, Intermountain has been a pioneer in telemedicine, which has proven especially valuable during the COVID-19 pandemic. The adoption of telehealth has allowed for effective patient management while reducing the need for in-person visits, thereby maintaining continuity of care and reducing exposure risks.
Conclusion
Through this exploration of predictive analytics in revenue cycle management (RCM), it becomes evident that the integration of data-driven strategies offers a profound opportunity for healthcare providers to enhance operational efficiency and patient outcomes. By leveraging the power of predictive analytics, organizations can navigate the complexities of revenue management, from improving claim submission accuracy to proactive denial management and personalized patient billing. These capabilities not only enhance financial performance but also contribute to a more efficient, patient-centered healthcare system. Companies like Wenour have showcased the tangible benefits of adopting such advanced technologies, marking a significant shift towards more sustainable and effective healthcare management practices.
As we conclude, it’s clear that predictive analytics stands as a transformative force within the healthcare industry, driving improvements across multiple facets of patient care and operational management. The success stories of Geisinger Health System and Intermountain Healthcare further underscore the potential of data analytics to revolutionize healthcare delivery and financial management. For healthcare providers aiming to stay at the forefront of innovation, especially those like Wenour, the adoption of predictive analytics in revenue cycle management is not merely an option but a necessity. As the healthcare landscape continues to evolve, embracing these technologies will be crucial for improving patient outcomes and achieving financial stability, setting a new standard for excellence in healthcare.
FAQs
1. How crucial are predictive analytics in managing revenue?
Predictive analytics are vital for revenue managers as they enable the optimization of pricing strategies through data-driven insights. By utilizing predictive analytics, revenue managers can implement dynamic pricing, adjusting prices based on real-time variations in demand, supply, and competition.
2. What role does data analytics play in the revenue cycle?
Data analytics is essential in Revenue Cycle Management as it provides actionable insights that drive decision-making and strategy formulation. It helps in identifying revenue trends, predicting future outcomes, monitoring performance metrics, and optimizing workflows.
3. What does prescriptive analytics entail within the revenue cycle?
Prescriptive analytics is crucial for making informed decisions in revenue cycle management. It aids in determining the most effective approaches for patient communications regarding unpaid bills and optimizing the claims submission process.
4. What are the key steps in the predictive analysis process?
The predictive analytics process involves several key steps:
- Define your project’s objectives: Determine what you aim to achieve.
- Collect your data: Assemble all necessary data in one place.
- Clean and prepare your data: Ensure the data is accurate and usable.
- Build and test your model: Create predictive models and test them for effectiveness.
- Deploy your model: Implement the model in a real-world scenario.
- Monitor and refine your model: Continuously assess and improve the model’s performance.